Podcast
Bringing AI Into Medicine and Keeping It Safe
Apr 16, 2024

“We really need to think about different ways of how we implement these systems beyond just replacing whatever workflow used to exist.”
Steven Horng, MD, MMSc, FACEP
Beth Israel Deaconess Medical Center
Narrator: As artificial intelligence, or AI, takes off in the public sphere, what about medicine? The health care industry has been using some form of AI for decades, yet very recent advancements are upping the ante. Machines that instantly interpret large amounts of data and can learn and problem solve will be used to help providers deliver safer and better health care. At the same time, what are the clinical risks that this new technology presents and must be considered? The Harvard medical community’s malpractice insurance company, CRICO, recently considered these issues at an annual gathering of defense attorneys.
Dr. Horng: Well, thank you for the great introduction, and it’s really a pleasure to be here.
Narrator: Dr. Steven Horng is a keynote speaker on the topic of AI. Dr. Horng practices as an emergency medicine physician at Beth Israel Deaconess, where he’s also the clinical lead for machine learning. In that role, he leads the deployment of this technology throughout the hospital in an effort to help direct clinical care. Dr. Horng is also board certified in Clinical Informatics, which is the science of how data, information, and knowledge can be used to improve the delivery of health care. And he currently serves as the director of the ACGME Fellowship in the subspecialty. Dr. Horng is a co-founder of Layer Health, an artificial intelligence startup out of MIT, which is focused on using the power of large language models to improve clinical care.
Dr. Horng: This has been the last decade or more of my life of really thinking about machine learning and how we can improve care, and so really great that the rest of the world picked that up and it’s a thing.
Narrator: Dr. Horng began to describe some of the key ways AI is applied to medicine and how providers can think about the clinical risks. To answer the question “why now,” Dr. Horng began with a quick look at the recent evolution of Artificial Intelligence:
Dr. Horng: All right, so a quick walk down memory lane of what artificial intelligence has been, and there’s really three epochs that we’ve gone through; expert systems, statistical learning, and then the newest which is generative models. So really back early, back in the day, we used to have these things called expert systems, which are basically rules-based approaches. So thinking about e-mail, if you wanted to figure out if you had spam or not spam, you might just create some filters that said, well if the words Nigerian Prince were in your e-mail, it’s probably spam, or something about lottery winnings or other things, right? So you create a bunch of rules that kind of encapsulates some expert knowledge.
Narrator: In medicine, examples might be a diabetic patient with an infection getting a certain medication or a patient with an allergy getting alternative medication: decisions based on rules based on expert knowledge.
Dr. Horng: This is what happened in like the 60s or 70s with programs like MYCIN and others and that got us only so far. Fast forward, and we started to come up with statistical methods. Let’s take all the data that we collected. Now, if you remember, back in the day in Gmail or others, you would like kind of press and say this e-mail is spam, this e-mail is not spam, and they learned machine learning models to predict if things were spam or not spam. So, that’s an example of statistical learning where we take all the data that you had before and we train a model on it. Those would be things like logistic regression, linear regression, all the things that we do in biostatistics or statistics could generally be considered statistical learning in this area called supervised learning, where we know the thing that we want and then we build a model for it.
Narrator: Dr. Horng says that medicine has been in this stage of AI for the past couple of decades. But fast-forward to the most recent handful of years and things began changing rapidly.
Dr. Horng: And we started coming up with ways of thinking about how we could build these models without any labels. A good example of that—this is work that was done about five years ago, seven years maybe now—on generative models. So, ChatGPT was not the first. And what really made the difference was in statistical learning. We had all this data in the past and we tried to build models from it based on things that we wanted to predict. What generative model said was well, let’s try to build a model of the world of how to actually generate these things. In this example, they try to create a model which would generate peoples’ faces and you could give it things like, well, let’s make them older or younger, let’s increase or decrease their hairline, let’s make them more male or more female, add glasses or not glasses, beard, no beard, wavy hair. So, these are all different kind of axes that one might want to generate different types of images. And that really is what defines Generative Learning, in that we’re trying to create models which you can generate images, whether those are peoples’ faces, whether those are legal briefs, whether those are medical notes, and that’s been something that we’ve been kind of working on and really came to the foray of its own with ChatGPT, when it really got good enough, good enough to be better than just noise.
Narrator: What makes now different for health care and the rest of the world? According to Dr. Horng, some of the credit goes to video gamers.
Dr. Horng: So back in the 90s, if anyone remembered using speech recognition back then, it was pretty terrible, right? You would say something and it would be pretty awful and then eventually it got to Siri and it was pretty good. And, so, on the left-hand side, you could see log error, and the error rate was really, really bad, and then somewhere around 2014 or so it got better than humans. So whatever you dictated was better than a transcriptionist who would do the same thing. So, what made this possible? And when I do this it’s both for images and text, it’s all kind of the same, it’s data. And shallow neural networks are more or less just a representation of how your brain would work, right? You’ve got brain cells, you have neurons, they’re interconnected with one another. You use one neural pathway more than another that gets activated, you use one less and that gets deactivated. And that’s what a neural network is. So, we’ve been doing this since like the 60s or 70s, and all we could do was build these really shallow networks; we just couldn’t more than that.
Narrator: The reason the industry was stuck was simple. We just didn’t have enough computer power.
Dr. Horng: So, even though your brain has, like, you know, billions of neurons, we could do just one layer instead of, like, you know, the 1000 layers that you actually need. Lo and behold, we had a bunch of gamers, and we built these things called GPUs, and we suddenly, because we wanted to play video games, came up with enough compute power to actually power these problems. And that really was the genesis. One, we started collecting enough data; and two, we had enough compute power to make these really deep networks, not one layer but, you know, tens of layers.
Narrator: With enough power and enough data, new possibilities emerged. In clinical care the diagnostic process is one place that can benefit. Diagnostic errors are the biggest source of malpractice cases, according to the national Candello database of medical professional liability claims.
Dr. Horng: So, this is a study that was done around identifying diabetic retinopathy. So, if you look at the back of someone’s eye, you want to figure out if they might have some complication of diabetes and if you do, you need more specialty care. And this is one of their early works—I believe this was at Stanford—that started to show that this was possible and it was that these types of models weren’t just good at identifying dogs, and chairs, and people, but could be used in exactly the same way to identify medical pathologies. Same thing with EKGs. If you have an Apple Watch these days, they’re actually pretty good in identifying A-fib and other arrhythmias, using exactly the same technology of using these deep learning models to identify arrhythmias. Just in the same way that you identify a chair, a person, or what have you.
Narrator: AI has been seeping into health care for a long time. An historical example is dictation. These days most clinicians use speech recognition apps to dictate notes. Dr. Horng says there is certainly more to come that will similarly impact everybody.
Dr. Horng: In the last year or so, you’ve seen ChatGPT really come out. So what things are going to change? So, you know, right now some of the risks.; we do dictation. Almost everyone does dictation; Nuance, Dragon Medical One, MModal, Siri, et cetera, are commonly being used almost by every clinician in how they dictate notes. But we know there’s problems. So, this is a study done over at the Brigham by my friends Lezo and Fiji Goss around looking at the speech recognition errors. If you ever read a medical note, you know we have this saying called "Dragonisms." They’re the most, like, ridiculous things because someone dictated this and didn’t really proofread it that well. You see in this study, there was a 7 percent error rate of which 5.7 percent of them were clinically significant, which is to say that it was clinically relevant information and had someone acted on what was actually written, harm would have happened. That’s 7.4 percent in the ED in this one study. If you look at further literature in radiology and others, that goes up to like 25 percent. So, a significant problem. Obviously, after you review it and you do it well, that drops down to .3 percent of which 6.4 percent are relevant. And so great technology timesaver but even this really mature technology, a technology that’s better than humans alone, still has significant potential for harm.
Narrator: Another well-known risk from an early form of AI resulted in a Joint Commission Sentinel Event Alert around 2011. The warning followed a newspaper report that pinned 200 deaths over a five-year period to “alarm fatigue.” Providers get so many audible and visual alarms doing their work that are often not valid for the individual patient, that they ignore the alarms or override them. AI modalities need to subtract from, not add to, the numbness of multiple alarms.
Dr. Horng: There’s a really famous case in San Francisco at UCSF, in their pediatrics, where someone got orders of magnitude more drug than they were supposed to because they kind of just like agreed with whatever the system said. And so, we see that all the time where if you just keep alerting people, people just become numb to it, which really goes counter to a lot of how patient safety works. You have a Sentinel Event, you do a Root Cause Analysis, and you figure out this is the thing if we only change, if we only stop them, then this event wouldn’t have happened. Well, it’s a really rare event and now you have the same alert, alerting on every patient and now everyone just ignores that alert.
Narrator: One idea for reducing alarms but still giving clinicians real-time guidance is called “nudging.” The clinicians see options highlighted in a color telling them the suggested option when ordering a study, but giving the individual the chance to choose a different option. Same with dictating encounter notes. Dr. Horng suggested a bit of risk management as well: careful documentation of what the technology prompted or nudged the clinician to say or do. Further complicating things is AI’s ability to have what they call “hallucinations.”
Dr. Horng: Hallucinations, absolutely. So, for those who don’t know, hallucinations are when ChatGPT just like, hallucinates things and it just makes up random facts or random cases, or legal opinions or things, right? It does that often enough. And so, it could be a problem, right? So, it could hallucinate that you have a child, or a dog, that you don’t have, you know, amongst other things.
Narrator: As for bias or hallucinations, Dr. Horng says that using one AI model to double-check another AI model shows promise for fixing, or at least minimizing those vulnerabilities.
Dr. Horng: It’s a tough one. If the model knew that it was going to be wrong, then it would have gotten it right in the first place. So, right now there’s a really good paper a couple of months ago by Microsoft, the researchers at Microsoft, where they said let’s take one model and use it to critique another model. So, you have one model that might be hallucinating, and so you ask a different model. So, say, you know, you take the one from Google, you take the one from Microsoft, and you take the Microsoft one and say, well, is the Google One right? Is it biased? Is it hallucinating? And by doing that you decrease your error significantly, right? So, let’s say you have a 5 percent hallucination rate at Google and a 5 percent hallucination rate at Microsoft, you use them to judge each other, and now you have a 5 percent times 5 percent, so that’s a .025 percent, something like that...you get a product of that, right? And so, you get you get a squared error effect and so that has been good at dramatically reducing it. That takes care of the hallucination problem. All of this is trained on the same data that is biased, and so how do you do that? Well, the data is biased. A lot of clinical knowledge and medicine is biased. So, how do you debias that? That is an open question that we don’t know.
Narrator: Another aspect of AI risk is overreliance on AI by providers. The advent of computer-aided breast imaging to help diagnose breast cancer, for example, is already common. They help clinicians catch more cancers that were otherwise missed. However, doctors started missing cancers they would have caught in the past, because the technology wasn’t perfect, and clinicians started expecting it to be.
Dr. Horng. Are we then missing things that we otherwise would have gotten, and what is the risk and liability around that? So, looking at these kind of prior examples, you’ve got dictation where there are clinically significant dictation errors, which we’re saying the physician should have proofread, but now there’s harm from it. You have the example of these bedside monitors where there was a fatal arrhythmia that was alarmed and someone ignored it and so, you know, there’s liability there. And then lastly, you have breast cancer diagnosis where you’ve made diagnoses that you otherwise wouldn’t have made, but now you’re missing some as well, and whose liability is that? And so all kind of open questions.
Narrator: With a new generation of machine learning health care applications, some old-fashioned risk management can be critical for patient safety. Whether it’s generative AI like ChatGPT or something newer that interprets an audio recording of the doctor/patient encounter and composes the notes without dictation, someone has to review and proofread. Added complexity comes from the tone of the AI-generated communication. Without review by the clinicians themselves, the wording may not be appropriate for a specific patient or condition. Placing a human at key points in the protocol to catch AI-related problems is important. An example like e-mail in-box management software shows the issues that providers and systems must address, especially communication with patients.
Dr. Horng: The problem is that one, that there’s a tone problem, there is the hallucination problem, and we know that physicians aren’t necessarily the best at proofreading, right? And I’m sure you’ve seen that in the charts that you read that we write but you’ll start to see this even more and more. So, what happens when a patient has a critical finding that needs to be acted upon and it was missed? What happens then? What we’ve seen with ChatGPT is it just misses things that are obvious. It gets really complicated things, which makes us think that it’s doing really well, and then it also misses things that are very, very obvious and are real problems. There are going to be huge misses and we’re going to blame the clinicians that, well, we told you, you should proofread it. Why didn’t you proofread it? Well because it looked okay and you press ‘send.’ Same way that we do with transcription, same way that we’ve done with medication orders and others, and so we really need to think about different ways of how we implement these systems beyond just replacing whatever workflow used to exist.
Narrator: Ultimately, some of the most important patient safety is to place humans in the path of AI, or to use AI as a back-up to human effort, as in the case of detecting breast cancer.
Dr. Horng: That’s one of the modes that this runs in, is using the model as a second annotator, as a second reviewer. So, the human does everything completely independently and let’s use the model to just double check, and let’s re-review anything where there’s a discrepancy. That’s definitely one of the modes where it’s useful. It gets a little trickier for some of the other use cases. Like, for example, the MRI for stroke use case, where a human can’t do it. And you come into this kind of philosophical realm where you need to start thinking about utility and social justice, where if the net effect of society is beneficial, then is that okay when you might have harmed one or, you know, a few patients when overall you would have saved a lot more? That sounds great from a philosophical perspective, not so great for the physician who’s on the losing side of that. Yeah. And so how does that work? I don’t know. I only have questions, not answers.
Narrator: We’ve seen dramatic advancement in just the past couple of years with AI. Where is it all going in the next five years?
Dr. Horng: Five years is a long time away. Five years is a very long time away. I think what you’re going to see is ChatGPT and other generative AI is going to improve. It’s not going to be exactly what was promised. What’s currently promised or envisioned is very optimistic. It ignores everything about things like workflow integration, all the other changes that need to happen, and it ignores the fact that it hallucinates, or other problems with it, right. And so, right now we’re at this really high peak of expectations in the Gartner Hype Curve. I think in five years we will definitely see some real changes around some of the monotony in medicine, where you could have taken a really smart college student, or high school student, and taught them to do a task. I think some of those tasks will start to be automated. I think the regulation landscape will change a bit because of the problems around liability, accountability, et cetera, and I think we’ll move have moved forward, not as much as you think. Thank you.
For Safety Net, I’m Tom Augello.
Commentators
- Steven Horng, MD, MMSc, FACEP
About the Series
We’ve got you.
Our Safety Net podcast features clinical and patient safety leaders from Harvard and around the world, bringing you the knowledge you need for safer patient care.
Episodes
Paying for Patient Safety: Solving an ROI Puzzle
Teleradiology Leads Virtual Care Risk in New Study
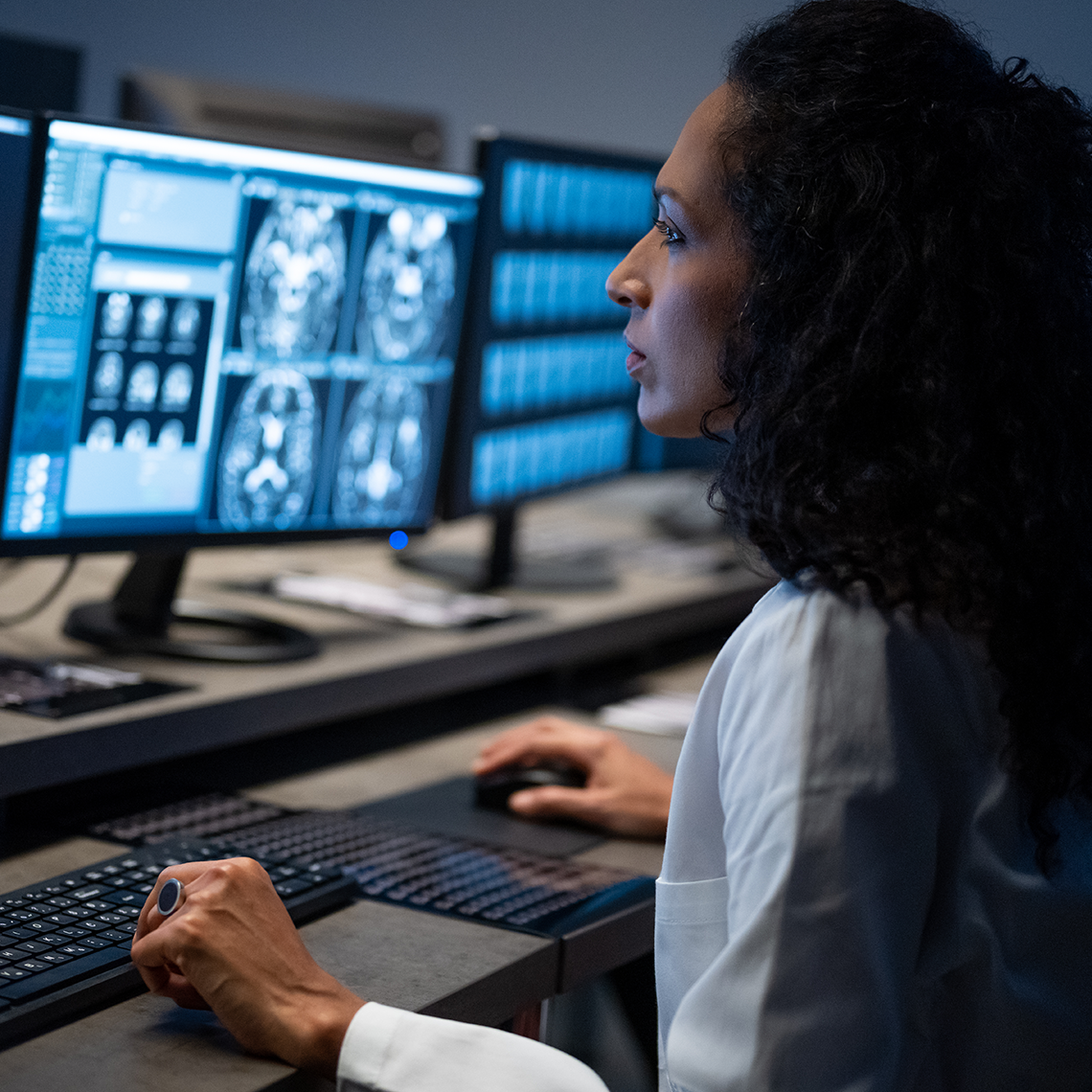
New Study Finds Outpatient Adverse Events Common, Often Preventable
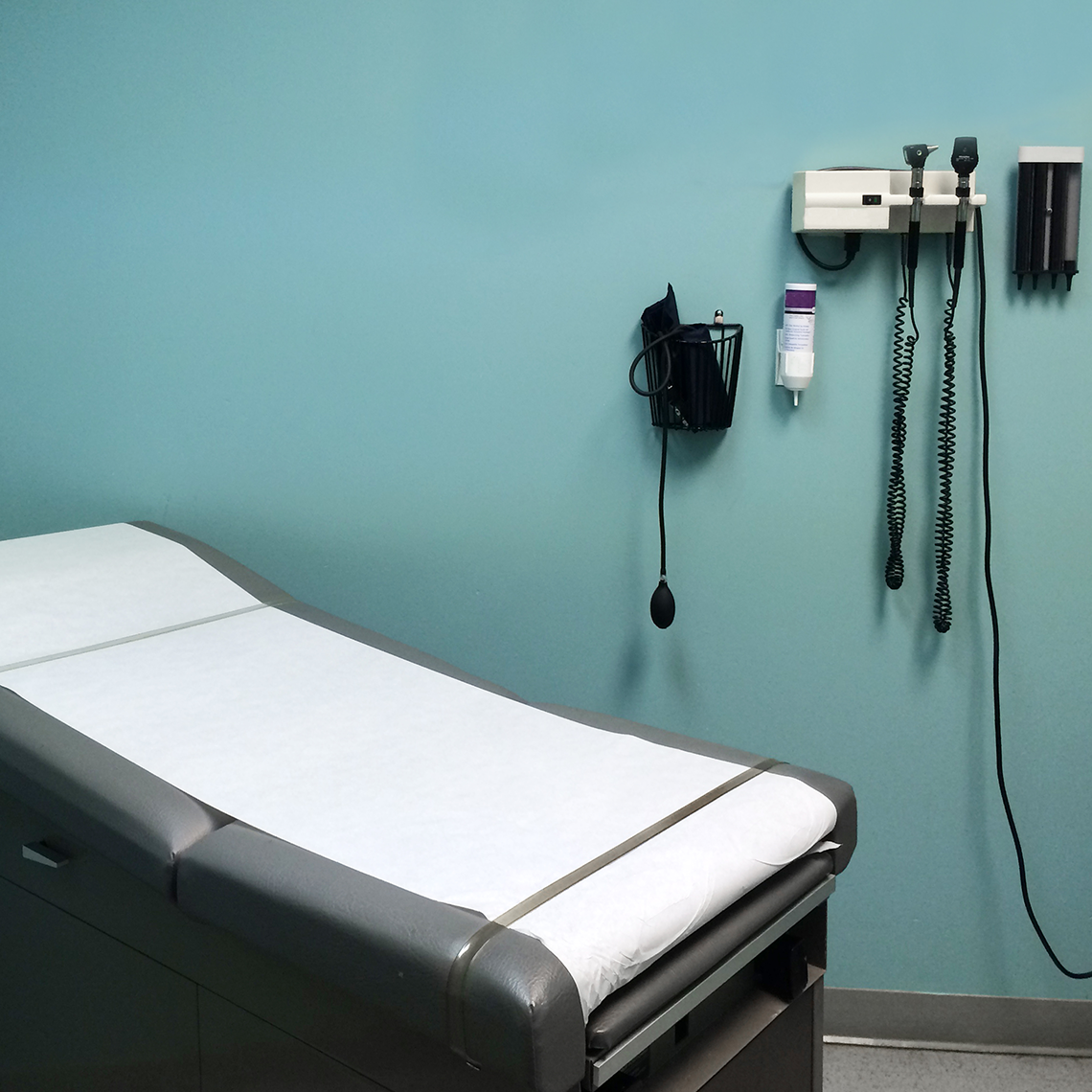
Taking the Pulse of a Clinician’s Interpersonal Skills
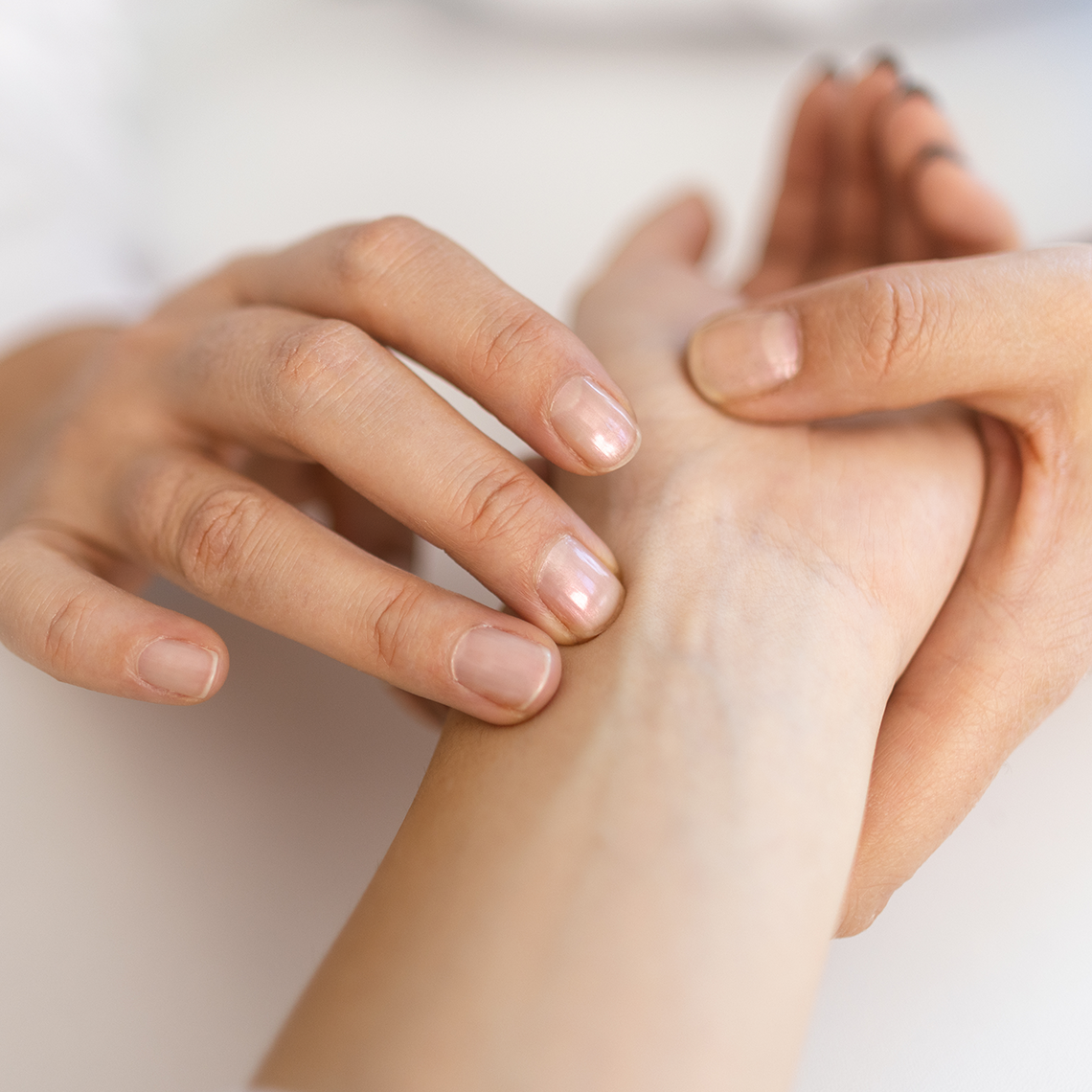